Mastering Robotic Skills in Real Visual Worlds through Model-based Reinforcement Learning
submit for ICRA2023, Paper video.
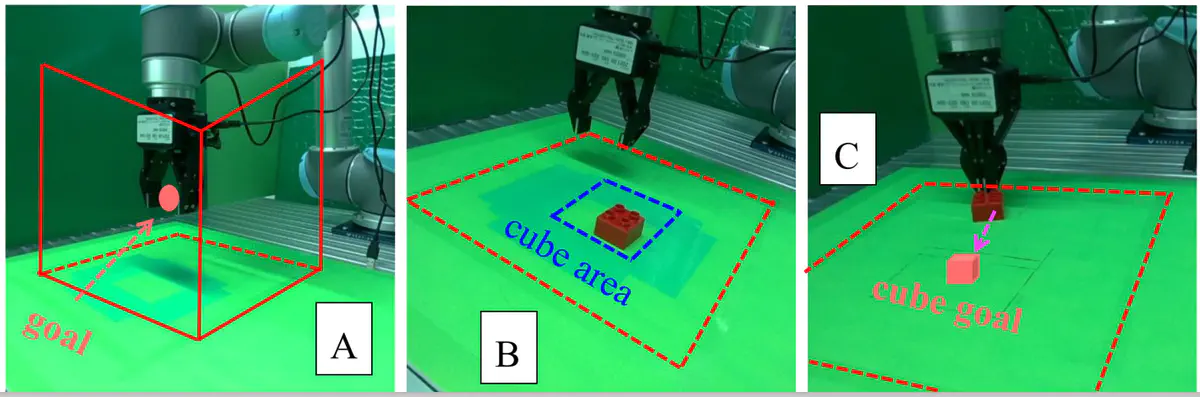
In the present work, a model-based reinforcement learning (RL), namely DayDreaming, is applied to quickly and accurately learn the robotic skills in the physical worlds only with the image observation. The model-based RL methods with the image observation can outperform the pure model-free RL frameworks in video games and robotic simulators, it is however rare to validate their advantages in the real worlds. With the aim of broadly applying the model-based RL algorithm and validating its effectiveness in the real-world scenarios, we directly utilize the physical robotic arm to learn diverse skills via the real-time interaction. To significantly reduce the real-world interaction time, a state transition model is created to generate the long-horizon simulated samples for the RL training. Since the only observation is the high-dimensional image which is too complicated to be directly used as the input and output of the transition model, we leverage contrastive learning to encode the image observation into a low-dimensional latent feature vector, which can exempt the commonly-used decoder of image reconstruction that might possibly cause object vanishing. The real comparison implementations with a robotic arm learning three different skills, reaching, positioning and pushing, demonstrate that our method distinctly outperforms another model-based RL algorithm and a model-free RL approach with respect of the learning efficiency and operation accuracy, which indicates a feasible way of widely applying the RL framework in the image-based real worlds.