Spatiotemporal Feature-Encoded Navigation for USVs in Unpredictable Maritime Scenarios
submit for IEEE Internet of Things Journal (IoT).
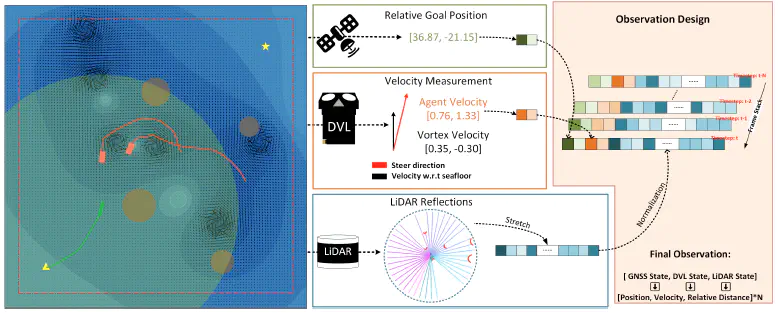
The paper proposes a robust collision avoidance framework for USVs navigating high-risk maritime environments without prior assumptions about surrounding obstacles. Current research typically assumes that known static obstacles and dynamic obstacles move in simplified patterns, failing to account for real-world conditions where human-operated vessels exhibit unpredictable and high-dynamic behaviors. To address this, we develop an innovative spatiotemporal feature-encoded navigation policy built on the implicit quantile network. The policy’s input is constructed using a sensor-based observation that integrates multiple sensors for environmental data collection, enabling a highly responsive and end-to-end navigation solution. Additionally, by employing convolutional neural networks (CNNs) to extract spatial features and utilizing a continuous-time closedform network to capture long-term motion dependencies, the proposed method effectively distinguishes between static and dynamic obstacles. The algorithm is compared with one reinforcement learning-based method and three classical methods. The results demonstrate its superior performance in navigating USVs through congested scenarios involving varying ocean flows, aggressive vessels, and static barriers.